In recent years, artificial intelligence (AI) has become a ubiquitous buzzword across industries. The potential of AI is vast and is transforming various industries from healthcare to finance, but it's not without its challenges. One of the major challenges of AI is the issue of transparency and interpretability. In many cases, AI models are considered 'black boxes,' making it difficult for humans to understand the decision-making process of these algorithms.
This is where Explainable AI (XAI) comes into the picture. XAI aims to make AI models transparent and interpretable to humans by providing insights into the decision-making process of these models. XAI is an emerging field that is gaining traction across various industries, as it enables humans to understand how AI systems arrive at their conclusions, which is especially important in sensitive applications such as healthcare and finance.
In this article, we will unpack the complex world of Explainable AI, exploring what it is, why it matters, and how it is transforming the way we interact with AI. We will also delve into the different techniques used in XAI and the challenges of implementing it in real-world applications.
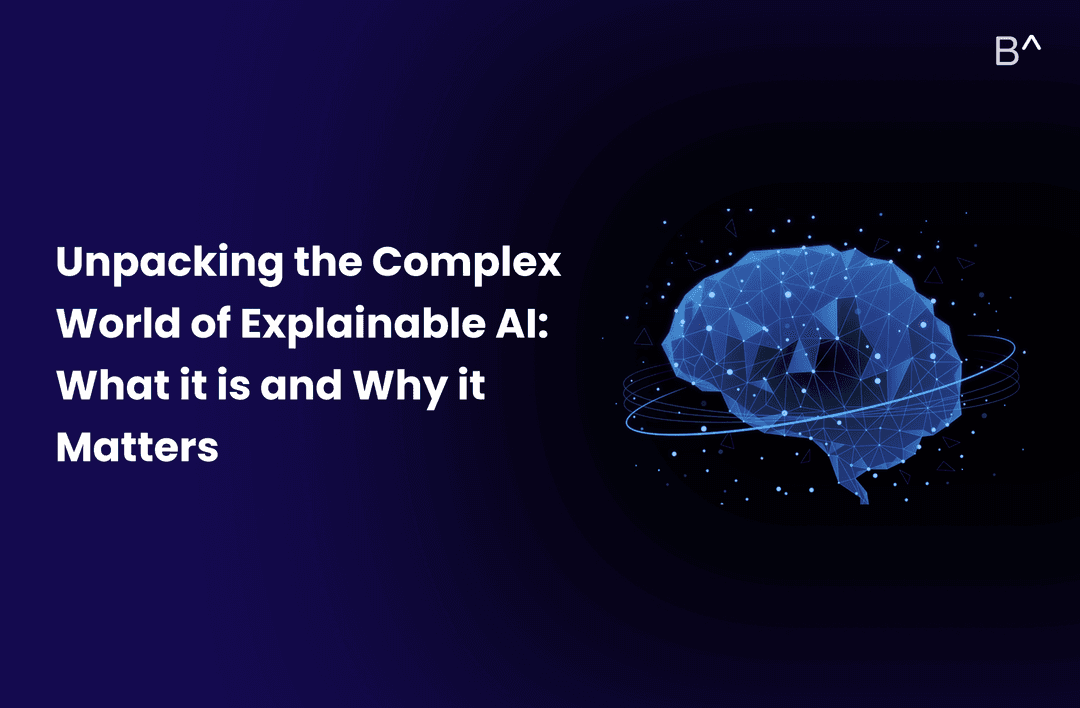
What Is Explainable AI?
Explainable AI (XAI) is an emerging subfield of artificial intelligence that focuses on developing machine learning models that can be easily understood and interpreted by humans. In simpler terms, it is the practice of creating AI models that can explain the reasoning behind their decisions or predictions. The goal of XAI is to make AI more transparent, trustworthy, and accountable, particularly in high-stakes domains like healthcare, finance, and criminal justice.
One of the challenges with traditional machine learning models is that they are often 'black boxes,' meaning that it can be difficult for humans to understand how the model arrived at its output. This lack of transparency can make it challenging to identify biases, errors, or potential ethical concerns within the model. XAI seeks to address this issue by developing models that are more interpretable and explainable, allowing humans to better understand the decision-making process and have greater trust in the model's outputs.
4 Principles of Explainable AI
1.Explain ability: AI systems must be designed to provide clear and concise explanations of their decision-making processes. This means that AI algorithms must be transparent, and the data they use to make decisions must be readily available for inspection.
2. Accountability: Explainable AI also promotes accountability in decision-making. With traditional AI models, it is difficult to assign responsibility for incorrect or biased decisions. Explainable AI models, on the other hand, can be audited and analysed to ensure that they are making decisions fairly and without bias.
3. Improved accuracy: Explainable AI models can also improve the accuracy of decisions. By providing clear explanations for how decisions are made, users can identify and correct any errors in the underlying data or algorithms. This can lead to more accurate and reliable results.
4. Better decision-making: Finally, explainable AI can lead to better decision-making. By providing clear explanations for how decisions are made, users can make more informed decisions based on the underlying data and analysis. This can lead to better outcomes and improved performance in a wide range of applications, from healthcare and finance to manufacturing and logistics.