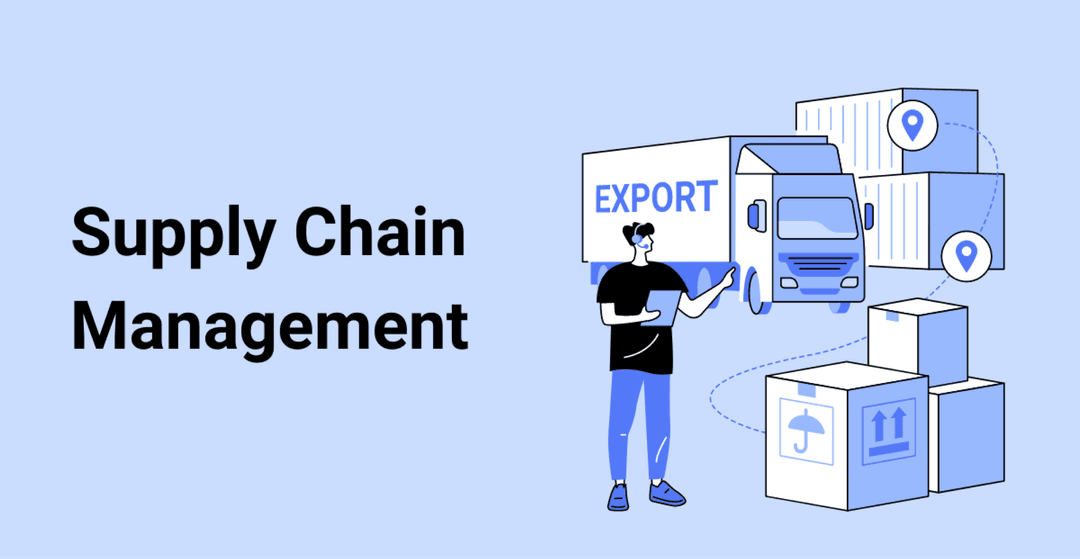
Summary
Machine learning-based forecasting has become an increasingly popular approach in supply chain management for its ability to improve forecasting accuracy. By leveraging historical data and other relevant information, machine learning algorithms can identify patterns and trends in the data and use them to make predictions about future demand, inventory levels, and other critical supply chain variables. This approach involves using various techniques such as regression analysis, time series analysis, neural networks, and random forest to analyse data and identify patterns that can be used to make more accurate predictions. By implementing machine learning-based forecasting in supply chain management, companies can better manage their supply chain, reduce costs, and improve customer satisfaction. It can also help companies adjust their production and inventory levels more accurately, minimize waste, and improve resource allocation. Overall, machine learning-based forecasting can be a valuable tool for supply chain managers to make more informed decisions and optimize their operations.
Machine Learning Techniques utilized for supply chain forecasting
Regression analysis: This technique involves analysing the relationship between variables, such as demand and price, to identify patterns and make predictions.
Time series analysis: This technique involves analysing data over time to identify trends and patterns in the data, which can be used to make predictions about future trends.
*Random forest: This technique involves creating multiple decision trees and using them to make predictions. Random forest is often used in supply chain forecasting to handle large datasets with many variables.
Supply chain practitioners usually use old-school statistics to predict demand. But with the recent rise of machine learning algorithms, we have new tools at our disposal that can easily achieve excellent performance in terms of forecast accuracy for a typical industrial demand dataset. These models will be able to learn many relationships that are beyond the ability of traditional statistical models. For example, how to add external information (such as the weather) to a forecast model.
We all know that historical data can provide valuable insights to improve future decisions in any business function. However, it is next to impossible to generate correct insights manually and even difficult to forecast accurately from such a method.
It is here that we need the help of advanced analytical tools which can dig through millions of historical data points and incorporate learnings which can help improve our future actions. Machine learning tools offer this capability to learn from historical data. Post their learning, machine learning models can be deployed for generating forecasts as well as prescribing actions such as setting inventory levels and deciding optimum production quantity.
Overall, machine learning-based forecasting can help supply chain managers make more accurate predictions about demand, inventory levels, and other key variables. This can help them better manage their supply chain, reduce costs, and improve customer satisfaction.