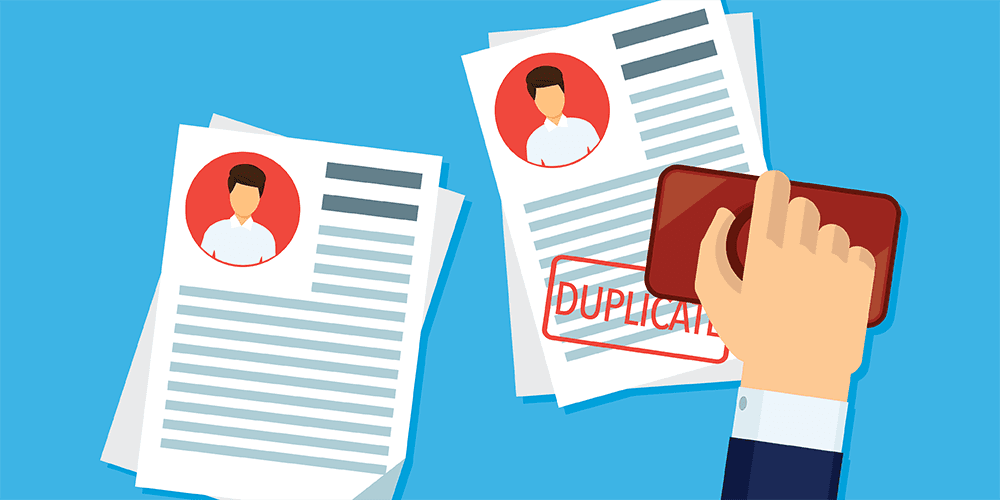
Summary
Database data duplication can have serious negative effects on enterprises, including higher storage costs, lower data quality, and slower data processing speeds. It becomes challenging to effectively evaluate and make' decisions based on the data when duplicate entries are present in
They can also result in wasted storage space since the same information is kept more than once on several servers, hard drives, or tape libraries.
Duplicate records can also be problematic when it comes to data migration because they increase the size and complexity of the transfer, slowing it down and perhaps leading to data loss or corruption.
Companies can employ a variety of techniques, including data deduplication and data cleansing, to address concerns with data duplication. Data cleansing involves finding and fixing mistakes and inconsistencies in the data, whereas data de-duplication removes superfluous data by locating and deleting duplicate information.
Businesses may have serious issues as a result of data duplication in databases, including higher storage costs, poorer data quality, and less effective data processing. To lower duplicate rates and increase the accuracy and efficiency of their data management processes, businesses should develop data quality protocols. Businesses may more efficiently identify and remove data duplication with the help of cutting-edge technologies like AI and machine learning, which will improve decision-making and result in cost savings.
Data duplication: the problem of plenty
When the same data is stored in many places, unwanted redundancy called data duplication results. Businesses that manage big amounts of data from numerous sources frequently run into this problem. Data duplication can raise storage costs, decrease data quality, and make data processing less effective.'The task of locating and deleting duplicate records is one of the key difficulties associated with data duplication. Conventional data deduplication techniques can take a lot of time and might not solve the problem completely. The process of locating and eliminating duplicate data can be automated with the use of cutting-edge technology like artificial intelligence (AI) and machine learning.
While machine learning models can be trained to precisely detect and delete duplicates, AI algorithms can evaluate patterns in data to find potential duplicates. This can assist firms in lowering storage expenses, enhancing data quality, and boosting data processing effectiveness.
In today's data-driven economy, data duplication is a significant difficulty for organisations. Yet, organisations can more effectively handle this issue and optimise their data management procedures by using cutting-edge technology like AI and machine learning.
Leveraging AI to avoid duplication
Data duplication is a significant challenge that businesses must overcome if accurate and effective data analysis is to be achieved. Fortunately, artificial intelligence (AI) can help detect and remove duplicate data in large datasets, lowering storage costs and increasing data processing efficiency. One of the most effective ways to use AI for data de-duplication is through machine learning algorithms. These algorithms can be trained on large datasets to identify duplicate records accurately, even if they are not exact matches. This method reduces the possibility of errors and increases the efficiency of the data de-duplication process.
Natural language processing (NLP) algorithms are yet another way to use AI to avoid data duplication. Possible duplicates can be identified based on context and meaning by analysing unstructured data, such as text, with NLP algorithms. This method can help to reduce the possibility of duplicate data in large databases, reduce storage costs, and improve data analysis quality. Furthermore, NLP algorithms can assist businesses in identifying and eliminating duplicates that are not easily identifiable using traditional methods, resulting in more accurate data analysis.
For businesses looking to optimise their data management operations, AI-powered data deduplication solutions are becoming increasingly important. Companies can improve data quality, increase data processing efficiency, and lower storage costs by using AI to identify and remove duplicate data. As the volume of data grows, AI-powered data deduplication solutions will become critical for businesses looking to stay competitive and extract the most value from their data.
Choosing a solution
1. Distance-based de-duplication:
A data de-duplication technique called distance-based deduplication uses mathematical techniques to assess how similar two sets of data are. A distance metric between two data sets is calculated, and duplicates are found using a predetermined threshold of similarity. This approach compares one set of data to another after transforming the original data into a mathematical representation, like a vector or hash value. Using a distance metric, such as cosine similarity, Euclidean distance, or Jaccard distance, the similarity between the two data sets is calculated. The data sets are regarded as duplicates and one of them may be deleted if the similarity is greater than a predetermined threshold.
The fact that distance-based deduplication may be used on both structured and unstructured data makes it beneficial for data sets of various sorts and formats, which is one of its main advantages. Also, this approach can spot duplicates even when they are not exact matches, which is useful when the data contains noise or has minor differences. However in order to function well, distance-based deduplication may need a sizable amount of processing power and be computationally expensive. Also, it calls for the declaration of a similarity threshold, which for some datasets can be difficult to establish. Despite these drawbacks, distance-based deduplication is nevertheless a useful technique for managing data and is used extensively across numerous industries.
2. Active-learning method:
Machine learning algorithms are used in the active-learning approach of data deduplication to find and eliminate duplicate data. To iteratively find and eliminate duplicates from a data set, a combination of human oversight and machine learning is used. In this procedure, duplicates are first found by a human expert who first examines a sample of the data set. This data is then used by the machine learning algorithm to find further data set duplicates.
The machine learning algorithm delivers duplicates to the human expert for confirmation as it finds them. The algorithm repeats this procedure until it has found as many duplicates as it can. The active-learning approach is particularly helpful in situations where data sets are too big to manually check for duplication. It can handle both structured and unstructured data.
As it combines the knowledge of human specialists with the strength of machine learning algorithms, the active-learning method has the potential to be more accurate than other deduplication methods. It also supports complex data sets with a variety of formats and architectures. Unfortunately, the active-learning approach necessitates a lot of human interaction, which can be time-consuming and expensive. To be effective, it also needs a well-built machine learning model. Despite these drawbacks, the active-learning approach is nonetheless a useful tool for managing data and is frequently employed in sectors like healthcare and finance.
Conclusion
AI has become a potent technique for data deduplication in recent years. AI is well adapted to the process of deduplication since it can manage vast volumes of data and recognise duplicates even when they are not exact matches. We can anticipate seeing more complex and efficient techniques for finding and deleting duplicate data as AI continues to develop. Ultimately, the application of AI for data deduplication can help firms save time, cut expenses, and increase the accuracy of their analyses.